A lire sur: http://searchdatamanagement.techtarget.com/feature/Data-quality-assessment-requires-business-ties-and-a-human-touch
Laura Sebastian-Coleman
Laura Sebastian-Coleman, the author of Measuring Data Quality for Ongoing Improvement: A Data Quality Assessment Framework, is a data quality architect at Optum Insight, which provides analytics, technology and consulting services to health care organizations. In this interview, she discusses her role in leading the creation of Optum Insight's Data Quality Assessment Framework (DQAF) and offers insights for organizations that are working to improve their data quality strategy.
What was your role in creating the DQAF? And can you give a little background on its development and how it has worked so far?
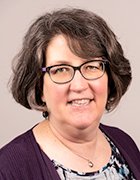
Laura Sebastian-Coleman: In 2009, I led the team of people at my company who created the original version of the DQAF. We wanted to define an extensible and practical approach to data quality measurement. We started by defining the dimensions we would focus on. Then we answered a set of questions about what, how and why we would measure. What does it mean to measure the completeness -- or timeliness, validity, consistency or integrity -- of data at the beginning of data processing? During data processing? After processing? What should measurement results look like? How would measurements detect when the data was not in the desired condition?
Answering these questions, we described ways to measure within the dimensions of quality in the framework and at different points in the data lifecycle. We called these ways measurement types: repeatable patterns of measurement to be executed against any data that fits the criteria of the type, regardless of specific data content. For example, any measurement of validity for a single column follows the same pattern as any other measurement of validity for a single column. In its simplest form, the DQAF is a detailed, generic and technology-independent description of ways to automate the measurement of data quality. It describes data collection, processing and analysis, and includes a logical data model for the storage of results. Much of the DQAF is common-sense data management.
In practice, some measurement types have wider applicability than others. We have applied a core subset of them, which focus on the consistency of medical claim data, in several largedatabases. The measurements have detected unexpected changes in the data, which analysts then investigate. Having the measurements in place has reduced the incidence of recurring problems and helped to manage similar risks. It has also enabled us to discuss requirements for the quality of data upfront, as part of development projects.
You offer comprehensive explanations and definitions of terminology related to data and data quality in your book. Why do you think it's important to differentiate, for example, between data and information or data and knowledge? How do people's different perceptions of those terms affect the outcomes of data quality assessment programs?
Sebastian-Coleman: Language is our primary tool for interacting with each other, but it requires effort. Clear definitions allow people to identify differences and form consensus. When an organization is trying to get value out of its data, the risk of misunderstanding each other is intensified. Business and technical people have very different vocabularies and different assumptions about data and data quality.
The data quality strategy has to align with an organization's overall mission or it will not bring value.Laura Sebastian-Coleman, data quality architect, Optum Insight
I carefully defined the terms you note -- information, data, andknowledge -- because I want people to think more about data. The relationship between data, information, knowledge and, ultimately, wisdom is often depicted as a pyramid with data on the bottom and wisdom at the top. Thinking of data as the base of this pyramid, we often forget that to produce usable data takes both information and knowledge.
Take the concept of temperature. Before the thermometer was developed, people perceived hot and cold, but they did not have a way to express these. It took several hundred years and a lot of intellectual effort to develop the thermometer. Today, we always know the temperature outside. Packed into that data point is knowledge. Data is compressed knowledge, made through trial and error. People envision what data they need to answer questions. Then they create the instruments to collect data. They define what the data represents and what brings about that representation.
People's perceptions of what data is can interfere with improving data quality. Sometimes data consumers expect data to exist that does not exist. Or they expect the data to represent something other than what it represents. If they do not know enough about the data, consumers can easily dismiss its quality. Knowledge and data are intimately related.
In developing an effective data quality strategy, what are some of the biggest challenges businesses face? And what can they do to get started down the right path?
Sebastian-Coleman: Organizations depend on data, but few manage it the way they manage other assets. Now, decades into the information age, most organizations have tons of data and data processing systems. The sheer amount can be overwhelming. Knowledge of data and systems is often undocumented, so data can be hard to use.
MORE ON DATA QUALITY ASSESSMENT FROM LAURA SEBASTIAN-COLEMAN
Learn the breakdown of data measurement termsin part one of an excerpt from her book
Read about data profiling, data quality issue management and reasonability checks in the second part of the excerpt
Addressing such challenges requires strategy -- that is, an intentional plan for success. The goal of a data strategy cannot be to fix everything, but to fix the things that matter and establish better ways to manage data. It should answer one big question: What do we need to do to have the data we need, in the condition it needs to be in, to support the mission of our organization over time?
Answering this question requires, first, being clear about the organization's mission and overall business strategy -- then stating explicitly how data supports that mission and business strategy. The data quality strategy has to align with an organization's overall mission or it will not bring value. To be effective, the strategy also needs to be used. People need to refer to it when they make decisions.
What kinds of tools can help facilitate the data quality assessment process?
Sebastian-Coleman: Improving data quality is a multifaceted challenge that requires a variety of activities and tools, including those, like profiling engines, that enable organizations to assess and manage the condition of their data. Profiling tools increase the efficiency of data analysis by surfacing important characteristics of data in a fraction of the time it would take an analyst to find them. Similar assertions can be made about tools that cleanse data. Profiling usually is focused on the initial condition of the data. Few organizations have an ongoing profiling practice. I have not seen a tool that measures data quality in-line, by applying profiling functionality to data processing and storing results to facilitate evaluation of trends, as described in the DQAF.
Getting benefits from tools requires analysts who can assess the data in relation to specific expectations or requirements. Data analysts are the most important part of any data assessment. A tool can find characteristics that should be evaluated. But a person needs to apply knowledge to make the actual evaluation.
Aucun commentaire:
Enregistrer un commentaire